Observational Data: Exploring What It Is And Where It Can Be Useful
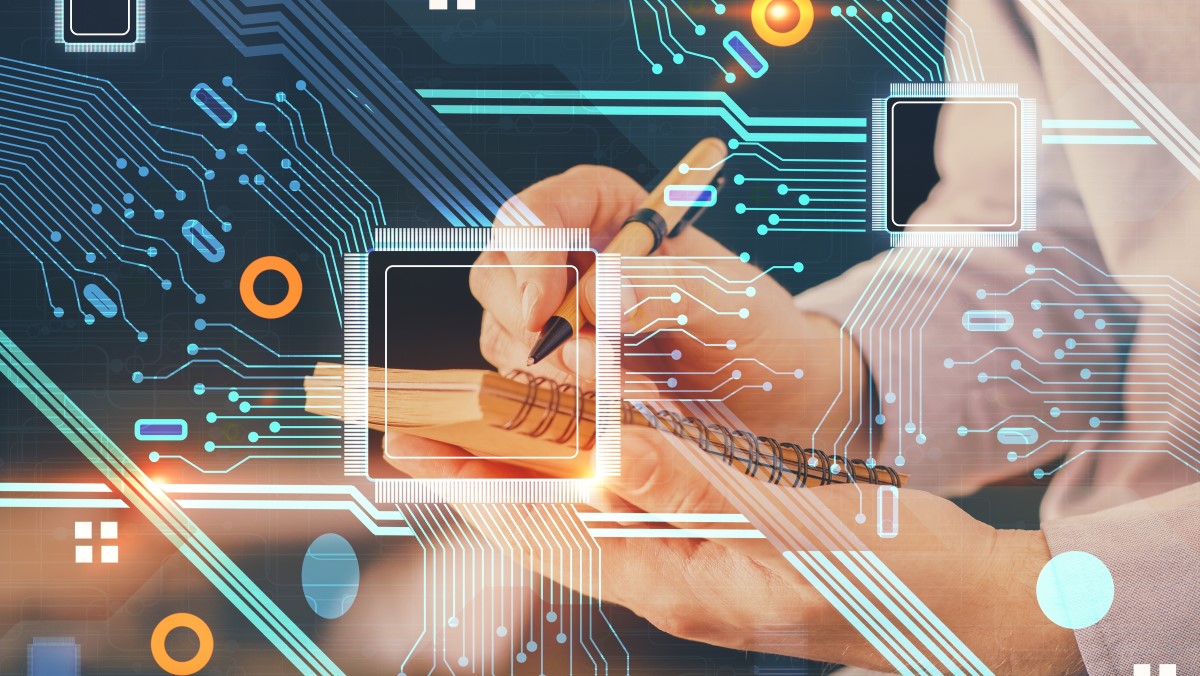
From business analytics to scientific research. The collection and analysis of data is right at the heart of today’s critical decision-making. Yet one particular type of data that holds a unique place in this domain is observational data.
In this blog, we will go on to investigate what this data is, the various types and the kinds of insights it can provide.
Types of observational data
There are two main types of observational data: structured and unstructured.
Structured
When it comes to structured observations, researchers follow a predefined plan or protocol to record specific aspects of a phenomenon. A highly controlled and standardised method, it helps to ensure all the data points are collected uniformly.
Good examples of structured observational data include surveys, rating scales and checklists. And it’s a data type that is often used in clinical practice fields like psychology, education and social sciences to study human behaviour and interactions.
Unstructured
Compared with the structured approach, unstructured observations are more flexible and open-ended. Researchers operate in a more relaxed manner, but observe events and behaviours as they occur, often without predefined categories or criteria.
This type of data collection is typically used in ethnographic studies, anthropology and for making naturalistic observations in ecology and biology.
Observational data insights
Depending on the research questions and methods used, observational data can provide many insights.
We’ve outlined some of the valuable insights that can be gained from this data below.
Understanding human behaviour
Observational data is particularly helpful for understanding human behaviour in different settings. It allows researchers to witness how people act in their natural environment, free from the interference and influence of surveys or experiments.
Pinpointing patterns and trends
It can help identify patterns and trends that might not be easily apparent when using other data collection methods. These patterns can help with making predictions or to inform decision-making.
Providing context
It offers a rich context to better understand events that may contain risk factors. Researchers can then better comprehend the circumstances and risk factors influencing any observed behaviours.
Quality assurance
Observational data is often used for quality assurance and process improvement in disciplines like healthcare and manufacturing. It’s valuable, as it helps identify issues and areas for improvement in real time.
Environmental research
Environmental scientists and ecologists use this data to study ecosystems, wildlife behaviour and the impact of human activities on the environment.
Market research
This data is also beneficial for market research, particularly in tracking consumer behaviour, preferences and trends. It’s helps optimise product placement, store layouts and marketing strategies.
Safety and security
When it comes to security, this data is ideal for monitoring and providing safety and security in a range of contexts from traffic management and surveillance systems to public spaces.
Techniques for gathering observational data
The unique insights that are possible with observational research, make this an extremely worthwhile approach for collecting observational data.
Depending on the nature of your research and its goals, there are a range of techniques available for gathering its data. These include:
Direct observation
This approach involves researchers physically observing the subject under study in its natural setting without interfering or influencing the observed behaviours. It’s a method that is typically used in fields such as psychology, anthropology and ecology.
Participant observation
Using this technique, researchers actively engage with the environment or control group they’re studying. Essentially, they become participants in the observation that allows them to gain an insider’s perspective.
It’s an approach that is common in ethnographic studies.
Structured observation
With this method researchers employ a predefined protocol or checklist to record specific behaviours or events. It’s an approach that helps ensure consistency and allows comparisons between observations to be made.
Unstructured observation
With unstructured observations researchers get more flexibility and can take notes on various behaviours and events as they occur. This method is particularly useful when the researcher wants to capture the complexity of a situation.
Video and audio recording
Video recording is another method that can help researchers capture observational data, enabling them to review and analyse behaviours and events in detail. It’s useful in various fields including education, healthcare and psychology.
Similar to video recording, audio recording can enable spoken interactions to be captured, which can be valuable for linguistic studies, communication research and qualitative data analysis.
Diaries and journals
With this approach, participants maintain diaries or journals to record their thoughts, experiences and behaviours. It's a method that allows researchers to build an in-depth understanding of individuals’ perspectives and experiences.
Remote sensing
In environmental and ecological studies, remote sensors and devices are often used, so that observational data can be more easily collected from a distance. This is especially beneficial for monitoring wildlife, weather and environmental conditions.
Challenges in dealing with observational data
Although observational data is valuable, it can pose a range of different challenges that researchers need to be able to address, if they’re to get the most out of this method. Here’s some areas to think about.
Insufficient control
In an ideal world observational data would provide the control found in experimental data, as without this it’s more challenging to establish causation or control for confounding variables.
Bias and confounding
Observational data can be prone to bias and confounding, which can lead to inaccurate or misleading results.
Data quality
As a result of issues like inaccuracies, missing data or measurement errors, the quality of this type of data can also vary significantly.
Ethical considerations
Gathering insights from individuals may pose ethical concerns, particularly if it involves the observation of people without their knowledge or consent.
Longitudinal data challenges
Longitudinal studies can be complicated due to the issue of participant churn and the need to handle data that’s collected over an extended period.
Large data volumes
Similarly, observational data collected over a long time can end up being huge and more challenging to manage, store and process as a result.
Data integration
It can also be challenging trying to integrate data from various sources, as this requires you to harmonize your data with different formats or units.
Complex analysis techniques
You may also require advanced statistical techniques for some observational data in order to address its various inherent challenges.
Interpreting your results
The complexity of real-world interactions can make it challenging to interpret your results. So, it’s essential to be cautious when you’re trying to draw conclusions from your data.
Replicability and generalisability
It’s important to note that replicating and conducting observational studies can be difficult, while it can be challenging to generalise your findings to broader populations or contexts.
Final thoughts
We hope you enjoyed this blog. And if you weren’t already familiar with the observational data approach, you now have a better idea of its benefits and how this can be applied to your business.
Whether it’s understanding human behaviour or studying the environment to trying to improve your processes. Whatever it is you’re trying to do, observational data can give you a unique perspective that complements other data collection methods. It also provides a richness of data, which you can use to answer complex questions or drive progress in range of different fields.
So, if you’ve never used the observational data approach before, there’s never been a better time to try it.